Self-Supervised Learning for Visual Obstacle Avoidance: Technical report
Synopsis
With a growing number of drones, the risk of collision with other air traffic or fixed obstacles increases. New safety measures are required to keep the operation of Unmanned Aerial Vehicles (UAVs) safe. One of these measures is the use of a Collision Avoidance System (CAS), a system that helps the drone autonomously detect and avoid obstacles.
Downloads
References
B. M. Albaker and N. A. Rahim. A Survey of Collision Avoidance Approaches for Unmanned Aerial Vehicles. In Technical Postgraduates (TECHPOS), 2009 International Conference for, 2009. doi:10.1109/TECHPOS.2009.5412074. https://doi.org/10.1109/TECHPOS.2009.5412074
Simon Baker, Daniel Scharstein, J. P. Lewis, Stefan Roth, Michael J. Black, and Richard Szeliski. A database and evaluation methodology for optical flow. International Journal of Computer Vision, 92(1):1-31, 2011. ISSN 09205691. doi:10.1007/s1126301003902. Data CC BYNC 2.0. https://doi.org/10.1007/s11263-010-0390-2
Michael Bloesch, Sammy Omari, Marco Hutter, and Roland Siegwart. Robust Visual Inertial Odometry Using a Direct EKF-Based Approach. In Intelligent Robots and Systems (IROS), 2015IEEE/RSJ International Conference on, pages 298-304. IEEE, 2015. ISBN 9781479999941. https://doi.org/10.1109/IROS.2015.7353389
Thomas Brox, Andrés Bruhn, Nils Papenberg, and Joachim Weickert. High Accuracy Optical Flow Estimation Based on a Theory for Warping. Computer Vision ECCV 2004, 3024:25-36, 2004.ISSN 03029743. doi:10.1007/9783540246732_3. https://doi.org/10.1007/978-3-540-24673-2_3
Richard Chen, Faisal Mahmood, Alan Yuille, and Nicholas J Durr. Rethinking Monocular Depth Estimation with Adversarial Training.arXiv preprint arXiv:1808.07528, 2018.
Xinjing Cheng, Peng Wang, and Ruigang Yang. Depth Estimation via Affinity Learned with Convolutional Spatial Propagation Network. arXiv preprint arXiv:1808.00150, 2018. URL http://arxiv.org/abs/1808.00150.
Marius Cordts, Mohamed Omran, Sebastian Ramos, Timo Rehfeld, Markus Enzweiler, Rodrigo Benenson, Uwe Franke, Stefan Roth, and Bernt Schiele. The Cityscapes Dataset for Semantic Urban Scene Understanding. In Proceedings of the IEEE conference on computer vision and pattern recognition, pages 3213-3223, 2016. ISBN 9781467388511. doi:10.1109/CVPR.2016.350. https://doi.org/10.1109/CVPR.2016.350
David Eigen and Rob Fergus. Predicting Depth, Surface Normals and Semantic Labels with a Common Multi-Scale Convolutional Architecture. In Proceedings of the IEEE International Conference on Computer Vision, pages 2650-2658, 2015. https://doi.org/10.1109/ICCV.2015.304
David Eigen, Christian Puhrsch, and Rob Fergus. Depth Map Prediction from a Single Image using a Multi-Scale Deep Network. In Advances in Neural Information Processing Systems 27, pages 2366-2374. Curran Associates, Inc., 2014.
Jakob Engel, Thomas Schops, and Daniel Cremers. LSDSLAM: Large Scale Direct monocular SLAM. Lecture Notes in Computer Science (including subseries Lecture Notes in Artificial Intelligence and Lecture Notes in Bioinformatics), 8690 LNCS(PART 2):834-849, 2014. ISSN16113349. doi:10.1007/9783319106052_54. https://doi.org/10.1007/978-3-319-10605-2_54
Jakob Engel, Jörg Stückler, and Daniel Cremers. Large-Scale Direct SLAM with Stereo Cameras. In Intelligent Robots and Systems (IROS), 2015 IEEE/RSJ International Conference on, pages1935-1942. IEEE, 2015. ISBN 9781479999941. doi:10.1109/IROS.2015.7353631. https://doi.org/10.1109/IROS.2015.7353631
Jakob Engel, Vladlen Koltun, and Daniel Cremers. Direct Sparse Odometry. IEEE Transactions on Pattern Analysis and Machine Intelligence, 2017. doi:10.1109/TPAMI.2017.2658577. https://doi.org/10.1109/TPAMI.2017.2658577
Christian Forster, Matia Pizzoli, and Davide Scaramuzza. SVO: Fast semidirect monocular visual odometry.Proceedings IEEE International Conference on Robotics and Automation, pages 15-22, 2014. ISSN 10504729. doi:10.1109/ICRA.2014.6906584. URL http://ieeexplore.ieee.org/lpdocs/epic03/wrapper.htm?arnumber=6906584. https://doi.org/10.1109/ICRA.2014.6906584
Christian Forster, Zichao Zhang, Michael Gassner, Manuel Werlberger, and Davide Scaramuzza. SVO: Semidirect Visual Odometry for Monocular and Multicamera Systems. IEEE Transactions on Robotics, 33(2):249-265, 2017. doi:10.1109/TRO.2016.2623335. https://doi.org/10.1109/TRO.2016.2623335
Adrien Gaidon, Qiao Wang, Yohann Cabon, and Eleonora Vig. Virtual Worlds as Proxy for Multi Object Tracking Analysis. In Proceedings of the IEEE conference on computer vision and pattern recognition, pages 4340-4349, 2016. https://doi.org/10.1109/CVPR.2016.470
Ravi Garg, Vijay B.G. Kumar, Gustavo Carneiro, and Ian Reid. Unsupervised CNN for Single View Depth Estimation: Geometry to the Rescue. In Bastian Leibe, Jiri Matas, Nicu Sebe, and Max Welling, editors, European Conference on Computer Vision, pages 740-756, Cham, 2016.Springer International Publishing. ISBN 9783319464848. doi:10.1007/9783319464848. https://doi.org/10.1007/978-3-319-46484-8_45
Andreas Geiger, Martin Roser, and Raquel Urtasun. Efficient Large-Scale Stereo Matching. In Computer Vision - ACCV 2010, pages 25-38, 2011. https://doi.org/10.1007/978-3-642-19315-6_3
Andreas Geiger, Philip Lenz, and Raquel Urtasun. Are we ready for Autonomous Driving? The KITTI Vision Benchmark Suite.Computer Vision and Pattern Recognition, pages 3354-3361,2012. https://doi.org/10.1109/CVPR.2012.6248074
James J. Gibson.The perception of the visual world. Houghton Mifflin, Oxford, England, 1950.
Clément Godard, Oisin Mac Aodha, and Gabriel J. Brostow. Unsupervised Monocular Depth Estimation with Left-Right Consistency. The IEEE Conference on Computer Vision and Pattern Recognition (CVPR), 2017. https://doi.org/10.1109/CVPR.2017.699
C. Goerzen, Z. Kong, and B. Mettler. A survey of motion planning algorithms from the perspective of autonomous UAV guidance, volume 57. 2010. ISBN 09210296. https://doi.org/10.1007/s10846-009-9383-1
Heiko Hirschmüller. Stereo Processing by Semiglobal Matching and Mutual Information ̈.IEEE Transactions on Pattern Analysis and Machine Intelligence, 30(2):328-341, 2008. https://doi.org/10.1109/TPAMI.2007.1166
Maximilian Jaritz, Raoul de Charette, Emilie Wirbel, Xavier Perrotton, and Fawzi Nashashibi. Sparse and Dense Data with CNNs: Depth Completion and Semantic Segmentation. arXivpreprint arXiv:1808.00769, aug 2018. https://doi.org/10.1109/3DV.2018.00017
Huaizu Jiang, Erik Learned Miller, Gustav Larsson, Michael Maire, and Greg Shakhnarovich. Self-Supervised Relative Depth Learning for Urban Scene Understanding. In Proceedings of the European Conference on Computer Vision (ECCV), pages 19-35, 2018. https://doi.org/10.1007/978-3-030-01252-6_2
Christian Kerl, Jurgen Sturm, and Daniel Cremers. Robust odometry estimation for RGBD cameras. In2013 IEEE International Conference on Robotics and Automation, pages 3748-3754. IEEE, may 2013. https://doi.org/10.1109/ICRA.2013.6631104
Georg Klein and David Murray. Parallel tracking and mapping for small AR workspaces.2007 6th IEEE and ACM International Symposium on Mixed and Augmented Reality, ISMAR, 2007. https://doi.org/10.1109/ISMAR.2007.4538852
Iro Laina, Christian Rupprecht, Vasileios Belagiannis, Federico Tombari, and Nassir Navab.Deeper depth prediction with fully convolutional residual networks.Proceedings 2016 4th International Conference on 3D Vision, 3DV 2016, pages 239-248, 2016. https://doi.org/10.1109/3DV.2016.32
Stefan Leutenegger, Simon Lynen, Michael Bosse, Roland Siegwart, and Paul Furgale. Keyframe-based visual-inertial odometry using nonlinear optimization. The International Journal of Robotics Research, 34(3):314-334, 2015. https://doi.org/10.1177/0278364914554813
Mingyang Li and Anastasios I Mourikis. High precision, consistent EKF based visual-inertial odometry. The International Journal of Robotics Research, 32(6):690-711, 2013. https://doi.org/10.1177/0278364913481251
Fangchang Ma and Sertac Karaman. Sparse to Dense: Depth Prediction from Sparse Depth Samples and a Single Image .arXiv preprint arXiv:1709.07492, 2017.
Fangchang Ma, Guilherme Venturelli Cavalheiro, and Sertac Karaman. Self supervised Sparse to Dense: Self supervised Depth Completion from LiDAR and Monocular Camera. arXiv preprintarXiv:1807.00275, 2018.
Julien Marzat, Sylvain Bertrand, and Alexandre Eudes. Reactive MPC for Autonomous MAV Navigation in Indoor Cluttered Environments: Flight Experiments, 2017 https://doi.org/10.1016/j.ifacol.2017.08.1910
Larry Matthies, Roland Brockers, Yoshiaki Kuwata, and Stephan Weiss. Stereo vision-based obstacle avoidance for micro air vehicles using disparity space. In 2014 IEEE International Conference on Robotics and Automation (ICRA), volume 9836, pages 3242-3249. IEEE, may 2014. https://doi.org/10.1109/ICRA.2014.6907325
Moritz Menze and Andreas Geiger. Object scene flow for autonomous vehicles. Proceedings of the IEEE Computer Society Conference on Computer Vision and Pattern Recognition, 07-12 June: 3061-3070, 2015. https://doi.org/10.1109/CVPR.2015.7298925
Javier Minguez, Florant Lamiraux, and Jean-Paul Laumond. Motion Planning and Obstacle Avoidance. Springer Handbook of Robotics, pages 1177-1202, 2016 https://doi.org/10.1007/978-3-319-32552-1_47
R. Mur-Artal and J.D. Tardós. ORBSLAM2: An Open-Source SLAM System for Monocular, Stereo, and RGBD Cameras. IEEE Transactions on Robotics, 33(5):1255-1262, 2017 https://doi.org/10.1109/TRO.2017.2705103
Raul Mur-Artal, JMM M M Montiel, and Juan D Tardos. ORBSLAM: A Versatile and Accurate Monocular SLAM System. IEEE Transactions on Robotics, 31(5):1147-1163, oct 2015. ISSN15523098 https://doi.org/10.1109/TRO.2015.2463671
Richard A Newcombe, Steven J Lovegrove, and Andrew J Davison. DTAM: Dense tracking and mapping in realtime. In 2011 International Conference on Computer Vision, pages 2320-2327.IEEE, nov 2011 https://doi.org/10.1109/ICCV.2011.6126513
Clint Nous, Roland Meertens, Christophe de Wagter, and Guido de Croon. Performance Evaluation in Obstacle Avoidance. In IEEE/RSJ International Conference on Intelligent Robots and Systems (IROS), pages 3614-3619, 2016. https://doi.org/10.1109/IROS.2016.7759532
Chris Olah, Alexander Mordvintsev, and Ludwig Schubert. Feature Visualization. Distill, 2017. https://doi.org/10.23915/distill.00007
Hung Pham, Scott A Smolka, Scott D Stoller, Dung Phan, and Junxing Yang. A survey on unmanned aerial vehicle collision avoidance systems. arXiv preprint,(arXiv:1508.07723), aug 2015. https://doi.org/10.48550/arXiv.1508.07723
Andrea Pilzer, Dan Xu, Mihai Marian Puscas, Elisa Ricci, and Nicu Sebe. Unsupervised Adversarial Depth Estimation using Cycled Generative Networks.arXiv preprint arXiv:1807.10915, 2018. https://doi.org/10.1109/3DV.2018.00073
Peter Pinggera, David Pfeiffer, Uwe Franke, and Rudolf Mester. Know Your Limits: Accuracy of Long Range Stereoscopic Object Measurements in Practice. In European Conference on Computer Vision, pages 96-111. Springer, 2014. https://doi.org/10.1007/978-3-319-10605-2_7
Matteo Poggi, Filippo Aleotti, Fabio Tosi, Stefano Mattoccia, and C V Jul. Towards realtime unsupervised monocular depth estimation on CPU. arXiv preprint arXiv:1806.11430, 2018. https://doi.org/10.1109/IROS.2018.8593814
Anurag Ranjan and Michael J. Black. Optical Flow Estimation using a Spatial Pyramid Network. In IEEE Conference on Computer Vision and Pattern Recognition (CVPR). IEEE, 2017. https://doi.org/10.1109/CVPR.2017.291
Martial Sanfourche, Vincent Vittori, and Guy Le Besnerais. eVO: a realtime embedded stereo odometry for MAV applications. In Intelligent Robots and Systems (IROS), 2013 IEEE/RSJ International Conference on, pages 2107-2114. IEEE, 2013. https://doi.org/10.1109/IROS.2013.6696651
Ashutosh Saxena, Sung H Chung, and Andrew Y Ng. Learning Depth from Single Monocular Images. Advances in Neural Information Processing Systems, 18:1161-1168, 2006.
Ashutosh Saxena, Sung H. Chung, and Andrew Y. Ng. 3D Depth Reconstruction from a Single Still Image. International Journal of Computer Visional of computer vision, 76(1):53-69, 2007. https://doi.org/10.1007/s11263-007-0071-y
Ashutosh Saxena, Min Sun, and A.Y. Ng. Make3D: Learning 3D Scene Structure from a Single Still Image. IEEE Transactions on Pattern Analysis and Machine Intelligence, 31(5):824-840, may2009. https://doi.org/10.1109/TPAMI.2008.132
Daniel Scharstein and Richard Szeliski. A Taxonomy and Evaluation of Dense Two Frame Stereo Correspondence Algorithms. International Journal of Computer Vision, 47(1/3):7-42,2002. https://doi.org/10.1023/A:1014573219977
Korbinian Schmid, Philipp Lutz, Teodor Tomić, Elmar Mair, and Heiko Hirschmüller. Autonomous Vision-based Micro Air Vehicle for Indoor and Outdoor Navigation. Journal of Field Robotics, 31(4):537-570, 2014. https://doi.org/10.1002/rob.21506
Nathan Silberman, Derek Hoiem, Pushmeet Kohli, and Rob Fergus. Indoor Segmentation and Support Inference from RGBD Images. In Andrew Fitzgibbon, Svetlana Lazebnik, Pietro Perona,YoichiSato, and Cordelia Schmid, editors, Computer Vision -ECCV2012, pages746-760, Berlin, Heidelberg, 2012. Springer Berlin Heidelberg. https://doi.org/10.1007/978-3-642-33715-4_54
Nikolai Smolyanskiy, Alexey Kamenev, and Stan Birchfield. On the Importance of Stereo for Accurate Depth Estimation: An Efficient Semi-Supervised Deep Neural Network Approach. arXivpreprint arXiv:1803.09719, 2018. https://doi.org/10.1109/CVPRW.2018.00147
Christoph Sprunk, Gershon Parent, Luciano Spinello, Gian Diego Tipaldi, Wolfram Burgard, and Mihai Jalobeanu. An Experimental Protocol for Benchmarking Robotic Indoor Navigation. In Experimental Robotics, pages 487-504. Springer International Publishing, 2015. https://doi.org/10.1007/978-3-319-23778-7_32
Christian Szegedy, Wojciech Zaremba, Ilya Sutskever, Joan Bruna, Dumitru Erhan, Ian Goodfellow, and Rob Fergus. Intriguing properties of neural networks. arXiv preprint arXiv:1312.6199, 2013. https://doi.org/10.48550/arXiv.1312.6199
Beau Tippetts, Dah Jye Lee, Kirt Lillywhite, and James Archibald. Review of stereo vision algorithms and their suitability for resource limited systems. Journal of Real-Time Image Processing, 11(1):5-25, 2016 https://doi.org/10.1007/s11554-012-0313-2
Vladyslav Usenko, Jakob Engel, Jörg Stückler, and Daniel Cremers. Direct Visual-Inertial Odometry with Stere oCameras. In Robotics and Automation (ICRA), 2016 IEEE International Conference on, pages1885-1892.IEEE,2016. https://doi.org/10.1109/ICRA.2016.7487335
Kevin van Hecke, Guido C.H.E. de Croon, Daniel Hennes, Timothy P. Setterfield, Alvar Saenz-Otero, and Dario Izzo. Self-supervised learning as an enabling technology for future space exploration robots: Iss experiments on monocular distance learning. Acta Astronautica, 140:1-9, 2017. https://doi.org/10.1016/j.actaastro.2017.07.038
Sudheendra Vijayanarasimhan, Susanna Ricco, Cordelia Schmid, Rahul Sukthankar, and Katerina Fragkiadaki. SfM-Net: Learning of Structure and Motion from Video. CoRR, abs/1704.0, 2017. http://dx.doi.org/10.48550/arXiv.1704.07804
Chamara Saroj Weerasekera, Thanuja Dharmasiri, Ravi Garg, Tom Drummond, and Ian Reid. Just-in-Time Reconstruction: Inpainting Sparse Maps using Single View Depth Predictors as Priors. arXiv preprint arXiv:1805.04239, 2018. https://doi.org/10.1109/ICRA.2018.8460549
Oliver J. Woodman. An Introduction to Inertial Navigation. Technical report, 2007.
Matthew D. Zeiler and Rob Fergus. Visualizing and Understanding Convolutional Networks. InDavid Fleet, Tomas Pajdla, Bernt Schiele, and TinneTuytelaars, editors,Computer Vision- ECCV2014, pages 818-833, Cham, 2014. Springer International Publishing. https://doi.org/10.1007/978-3-319-10590-1_53
Chuanxia Zheng, Tatjen Cham, and Jianfei Cai. T²Net: Synthetic-to-Realistic Translation for Solving Single-Image Depth Estimation Tasks. arXiv preprint arXiv:1808.01454, 2018. https://doi.org/10.1007/978-3-030-01234-2_47
Yiran Zhong, Yuchao Dai, and Hongdong Li. Self-Supervised Learning for Stereo Matching with Self-Improving Ability. sep 2017. http://dx.doi.org/10.48550/arXiv.1709.00930
Tinghui Zhou, Matthew Brown, Noah Snavely, and David G Lowe. Unsupervised Learning of Depth and Ego-Motion from Video. InCVPR, page 7, 2017. https://doi.org/10.1109/CVPR.2017.700
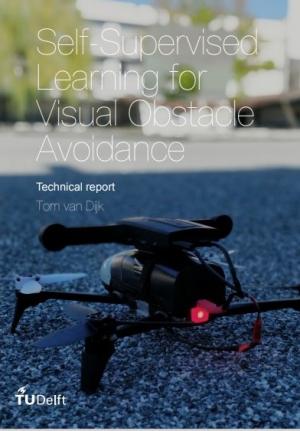